Framework for identification and ranking of difficulty factors when learning from imbalanced data
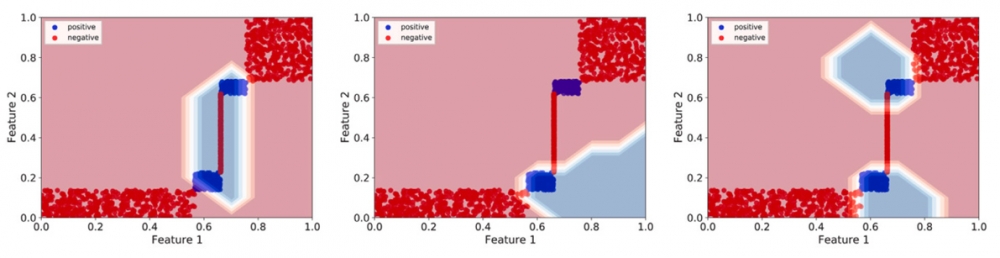
We have conducted a contemporary empirical study of the behaviour and performance of five well-known classifiers on a large number of imbalanced datasets exhibiting numerous combinations of the data intrinsic characteristics such as small disjuncts, class overlapping, noise and data rarity. The aim of the study is to identify and rank difficulty factors when learning from imbalanced data, depending on the type of classification algorithm used. To alleviate these problems, oversampling and undersampling procedures were tested and directions are given for selecting appropriate techniques when dealing with the problem of class imbalance.
Dudjak, M., & Martinović, G. (2021). An empirical study of data intrinsic characteristics that make learning from imbalanced data difficult. Expert Systems with Applications, 182, 115297. https://doi.org/10.1016/j.eswa.2021.115297
Data mining model for credit scoring based on feature selection and ensemble classifiers

We have proposed a hybrid data mining model based on a combination of feature selection procedures and an ensemble of classifiers. As part of the proposed model development methodology, five different feature selection algorithms were investigated, which were used with the support of voting procedures after the evaluation. Also, a new voting procedure has been proposed that achieves better performance than the existing ones. Several classification algorithms were combined into ensemble models using the proposed soft voting. Experimental data have shown that the proposed hybrid model based on the features obtained by soft voting and the proposed ensemble achieves very good performance and can be successfully used in the client creditworthiness assessment.
Nalić, J., Martinović, G., & Žagar, D. (2020). New hybrid data mining model for credit scoring based on feature selection algorithm and ensemble classifiers. Advanced Engineering Informatics, 45, 101130. https://doi.org/10.1016/j.aei.2020.101130
Nalić, J., & Martinović, G. (2020). Building a credit scoring model based on data mining approaches. International Journal of Software Engineering and Knowledge Engineering, 30(02), 147-169. https://doi.org/10.1142/s0218194020500072
Projekt: DATACROSS – Advanced methods and technologies in data science and cooperative systems